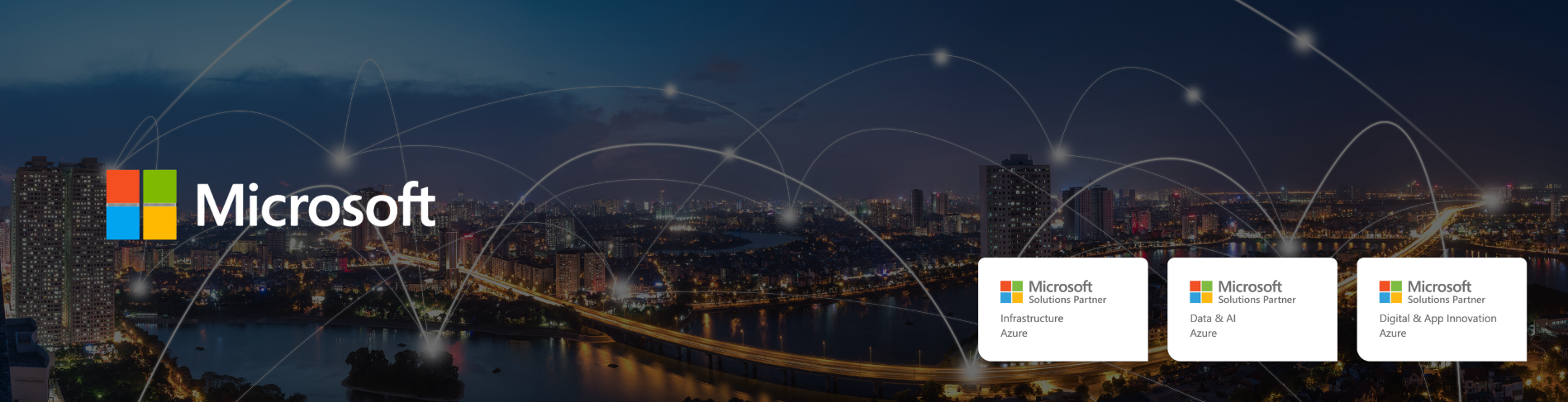
Overview
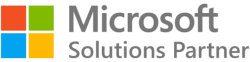
Highlights
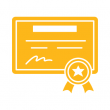
600+ Azure Certified Engineers
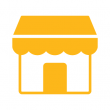
28+ Products on Azure Marketplace
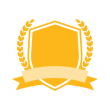
3 Solutions Partner Designations
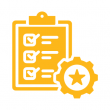
Scaling analytics and AI with enterprise IT
Supercharge Your Business with Generative AI
Transforming enterprises through finance intelligence
Solving Complex, Unstructured Problems
Resilient, Agile, Purpose-driven
Powering AI with responsible deployment
New dimensions of computing power
Derive deeper marketing insights
Environmental, Social and Governance Framework
Fractal Analytics Academy
Behavior Architects
Activating digital moments
Delivering continuous machine learning models & automation pipelines
Better Decisions Through Business Experimentation
Powering the next best experience
Innovators for computer vision
Empowering Business Through Automation
Bridging the gap between humans and machines
Automated Insights for Digital Evolution
Powering AI with Big Data
Catalyst for Growth
Consumer insights platform
Accelerated data to decision transformation